Table of Contents
The Halo Effect: How Preconceived User Perceptions Affect A/B Testing Results
A/B testing is an indispensable instrument for anybody in marketing, product management, or web development who cares about the quality of their digital assets or the level of interaction with their users. A/B testing allows for better-informed decisions by comparing two or more variants of a website, app interface, or marketing campaign, ultimately improving user experiences and conversion rates. The “Halo Effect,” on the other hand, is a psychological phenomenon that may gradually corrupt test findings and modify the accuracy of insights gained from trials, despite the apparent neutrality of A/B testing.
Top 3 A/B Testing Services
Here are the top 3 A/B testing services for email marketing:
- Selzy: Selzy is a convenient A/B testing service with a wide range of features to optimize the effectiveness of your email campaigns. Easily create and customize different email variants, and analyze their performance to find the most effective solutions.
- Google Analytics: Google Analytics is a popular and free web analytics tool that can be used for A/B testing email campaigns. It allows you to create link variants and track their interactions with recipients, as well as analyze performance metrics for better insights into audience engagement.
- UTM tags: UTM tags are parameters added to email URLs that allow you to track source, medium, and other details of clicks. They help measure the effectiveness of specific elements in your email campaigns, such as subject lines, images, CTAs, etc., and understand which variants perform better.
Using these tools, you may learn what parts of your email campaigns get the most clicks and conversions and then adjust your approach accordingly.
Understanding the Halo Effect
The Halo Effect is a kind of confirmation bias in which an individual’s preconceived notions about a person, brand, or product color their evaluation of its particular characteristics. This implies that customers may come into an A/B test with preexisting notions or prejudices regarding the brand in question, which may affect the results.
Imagine a well-known company running an A/B test between two versions of the checkout page on its website. Variant A’s design is a little old, whereas Variant B’s is contemporary and stylish. Users with a favorable “Halo” impression of the brand may be swayed by such sentiments to choose Variant B, despite the latter’s inferior performance, without even realizing it.
The Impact on A/B Testing Results
The Halo Effect can potentially obscure the actual effect of the modifications being evaluated, leading to inaccurate results. In the example shown earlier, if Variant B performs better than Variant A, it may not be exclusively owing to the enhancements made to its design; rather, it may be because people were favorably inclined to the brand prior to the beginning of the test. In addition, the Halo Effect may result in misleading negative findings, which is when a variant that has the potential to perform better is wrongly judged to be of lower quality. Even if the alternative choice has better functionality or content, users may have unfavorable opinions of the brand, which may cause them to avoid or disregard the alternative option.
Addressing the Halo Effect in A/B Testing
It is essential, in order to acquire correct insights from A/B testing, to first recognize and then address the Halo Effect. Listed below are some approaches that may be taken to lessen its effects:
Randomize Test Groups
A/B testing relies heavily on the notion of randomization. It entails allocating users to variants at random, with no consideration for personal preference. This guarantees that the test groups are really representative of the target population and that any inherent biases are spread out uniformly among them. This aids in reducing the Halo Effect’s influence on the outcomes of the exam.
Several techniques exist for implementing randomization, such as randomized cookies, server-side allocation, and automated A/B testing software.
Blind Testing
To conduct blind testing, often called single-blind testing, testers keep the identities of the test variations hidden from participants. In this method, customers have no idea whether they are experiencing Variant A or B. By keeping the test blind, we can make sure that participants’ expectations about the brand or product don’t affect their actions.
Tools and systems that make it easy to blindly show variants to users should be considered for use in conducting blind tests. These aids let consumers know exactly which version they are engaging with, eliminating any possibility of prejudice.
Control for Brand Perception
To correctly understand A/B test results, it might be helpful to know how consumers currently feel about the brand. It is useful to collect extra data about user attitudes and preferences toward the brand to better understand the influence of possible sources of bias on the test results.
This may be accomplished via questionnaires, interviews, or any other feedback techniques to ascertain the impressions, sentiments, and connections people have with the brand. This information and the A/B test results may provide light on how users’ preconceived notions about the brand affected their decisions throughout the experiment.
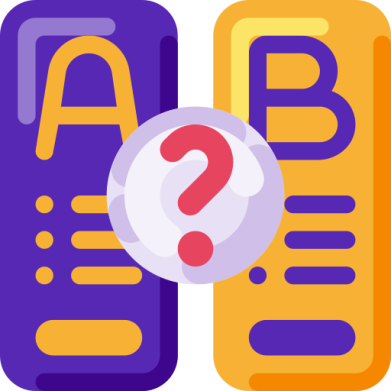
Larger Sample Sizes
In A/B testing, increasing the sample size is a powerful tool for mitigating the effects of subjectivity and background noise. A bigger sample size allows for a more accurate reflection of the user community and increases the likelihood of obtaining meaningful findings.
We can reduce the impact of the Halo Effect and other forms of individual bias by expanding our sample size. However, it is important to find a happy medium since excessively high sample sizes may be costly and time-consuming.
Long-Term Analysis
Given the often brief period of the majority of A/B testing, it is essential to consider the long-term performance of each variant. The transient alterations in user behavior may not accurately reflect the enduring impacts of the trials.
Research that follows participants over time, or “longitudinally,” may tell us whether or not observed differences between variations stick up over time. This helps understand the long-term impacts of the changes and ensures that short-term factors don’t skew the results.
Make Better Results with High-Quality A/B Testing
A/B testing is powerful when wielded with care and awareness of potential biases like the Halo Effect. By acknowledging the impact of preconceived user perceptions and employing appropriate methodologies to address them, businesses can unlock more accurate insights, make informed decisions, and ultimately create superior user experiences.
Share Your Thoughts